Summary
In this blog post, cross-published with the Montreal International Centre of Expertise in Artificial Intelligence (CEIMIA), Antoine Glory, Machine Learning Engineer and AI Project Coordinator at CEIMIA, explores current trends in the development of Generative AI. He examines how it is often applied across various technologies without sufficient consideration of its real needs, and evaluates the industry's decisions in light of the environmental and climate impact of these technologies.
Say you want to look up who Ada Lovelace was – and maybe you do, now! If we’re in 2020 there’s a 95% chance you will use Google or a similar search engine. Today, it is probable that your search engine will use some form of Large Language Model (LLM) to respond to your query. But LLMs don’t necessarily perform better and what you may not be seeing is the cost of these two queries. While they are both free for the end-user, one has a much larger environmental cost throughout its lifecycle, from rare earth materials, to water usage, model training, etc.
Such considerations also raise the question of decision-making mechanisms when using and deploying AI systems, as well as the impact of these choices on the environment. How can users make informed decisions when using AI tools? What are AI deployers’ sustainability considerations? How are regulations and interoperability mitigating or exacerbating AI’s impact on the environment?
Consumer overuse: between lack of awareness and tool appropriateness
While GenAI tools can offer innovative and promising solutions, it is not obvious that they are necessary and better than a traditional search engine. A trend we are witnessing is users resorting to big AI models for search when either no AI or smaller AI models are actually better tools to achieve the same outcome – a ChatGPT query consumes 10-25 times more electricity than a Google search query.
Large language models are only one of AI’s currently booming fields, so the same question applies to many more applications, such as image or video generation. On the other hand, AI providers are also often over-deploying it. With AI becoming ubiquitous, efforts to mitigate its environmental impact are very creditable, yet the best optimization could often simply be to not use AI at all.
Over-deployment of AI: a trend critically enabling environmental damages
It is an accepted rule that good product design should focus on outcomes rather than features – and yet so many home appliances and electronics focus on integrating new features, whether or not they’re essential. We are witnessing this trend with AI, most of the time motivated by a race to follow the latest hype. From online fast fashion recommendation systems to chatbots, industry seems to be putting AI everywhere.
Speaking at IEEE webinar on Tackling Sustainability Through the AI Lifecycle, David Rolnick, professor at McGill university, brings very relevant insights to this matter: ‘many students grew up thinking “I’m just building the technologies”, and then nobody ends up actually thinking about their impact, or at least nobody with the ability to change things’. This lack of reflection on broader impacts can be seen in the development of self-driving cars. Typically, such technologies are expected to exacerbate climate change, as they will lead to more driving by lowering the barrier to it, even if each mile driven becomes more efficient. However, the same technology can be used for more sustainable purposes such as facilitating public transportation, or enabling low carbon freight options, such as self driving buses and trucks.
Rolnick warns against relying on tech solutions alone, a mindset termed as “techno-utopianism,” noting “the most important solutions are not about tech-ing our way out, but about people-ing our way out,” highlighting how the human factor should not be overlooked when searching for solutions to address climate change challenges.
Sustainability considerations: their place in the decision making process when deploying AI
We can easily see the direct environmental impact of AI such as the compute infrastructure required to run AI systems. Yet, as Johannes Kirnberger, an OECD policy expert, reminds us, we cannot ignore the indirect impacts that spread across the entire life cycle from production to transport, operation stage, until the end of life in disposal and recycling. In an IEEE survey aiming at estimating environmental trade off when deploying AI, respondents indicated that organizations were mainly focused on energy and not so much on water consumption – even if early stages of the AI systems life cycle, such as mining, involve very water-intensive processes. Additionally, organizations sometimes build large data centers in remote places with high water stress (e.g. in deserts). Such decisions are little based on environmental costs and more closely aligned with financial costs and/or jurisdictional considerations related to data acquisition, transfer and storage.
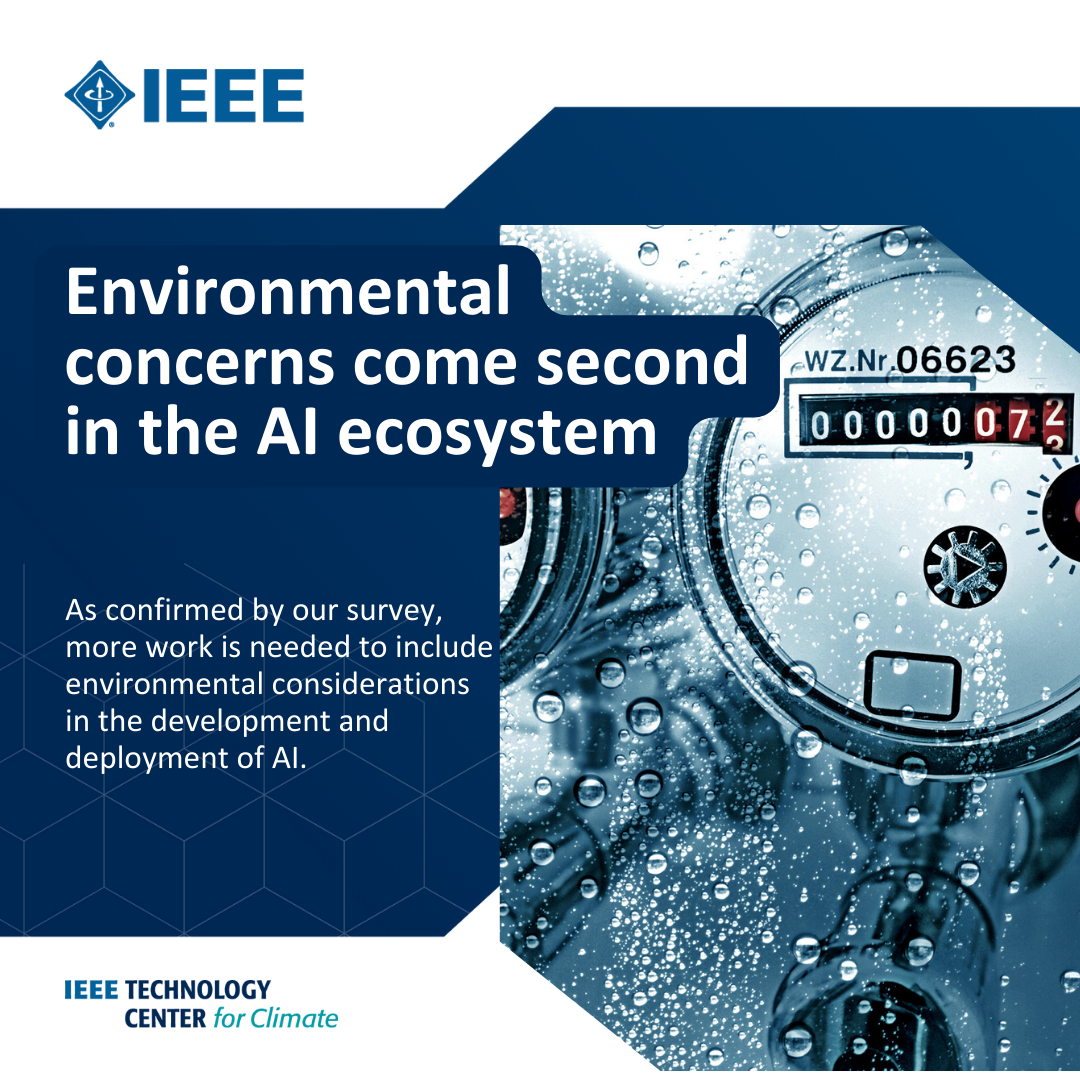
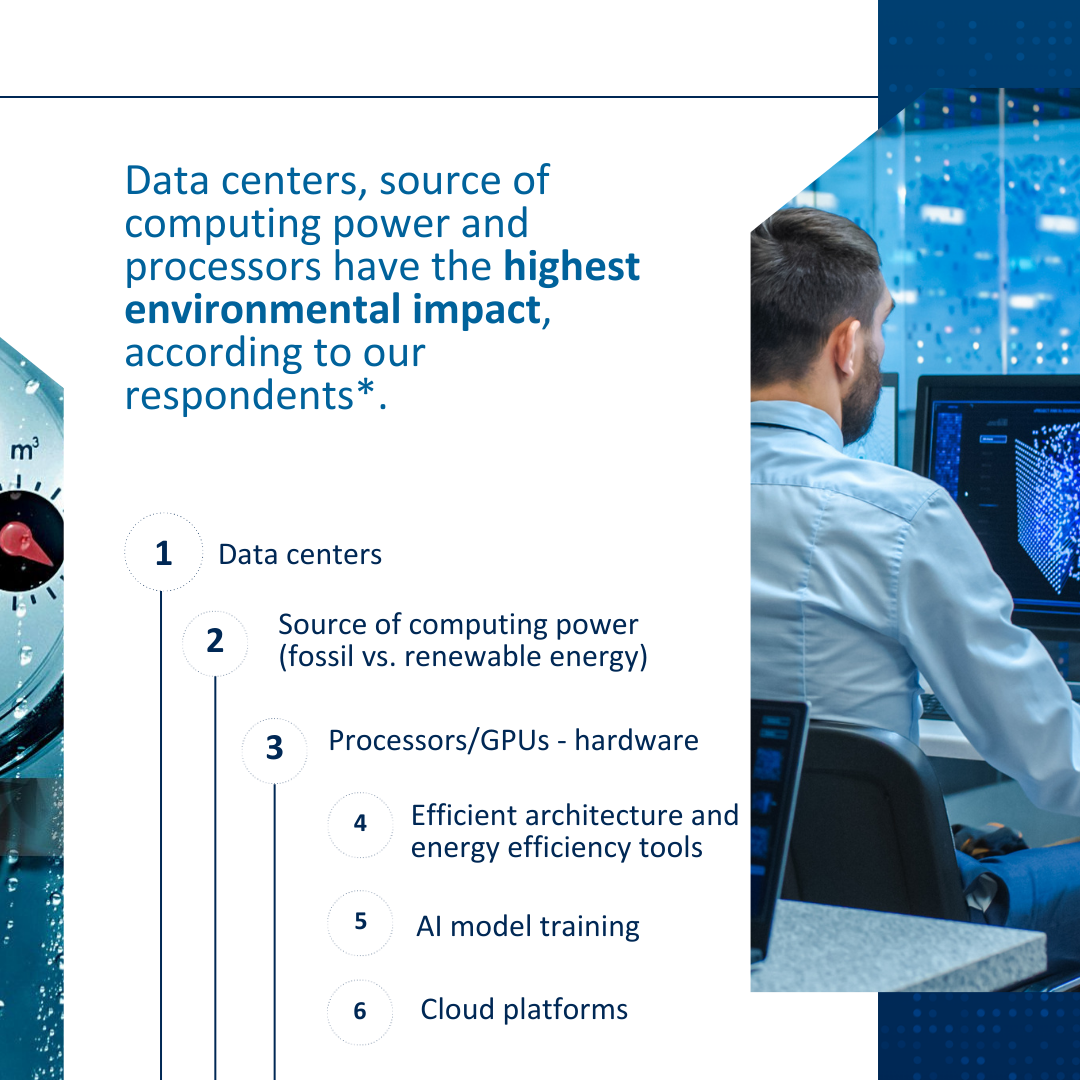
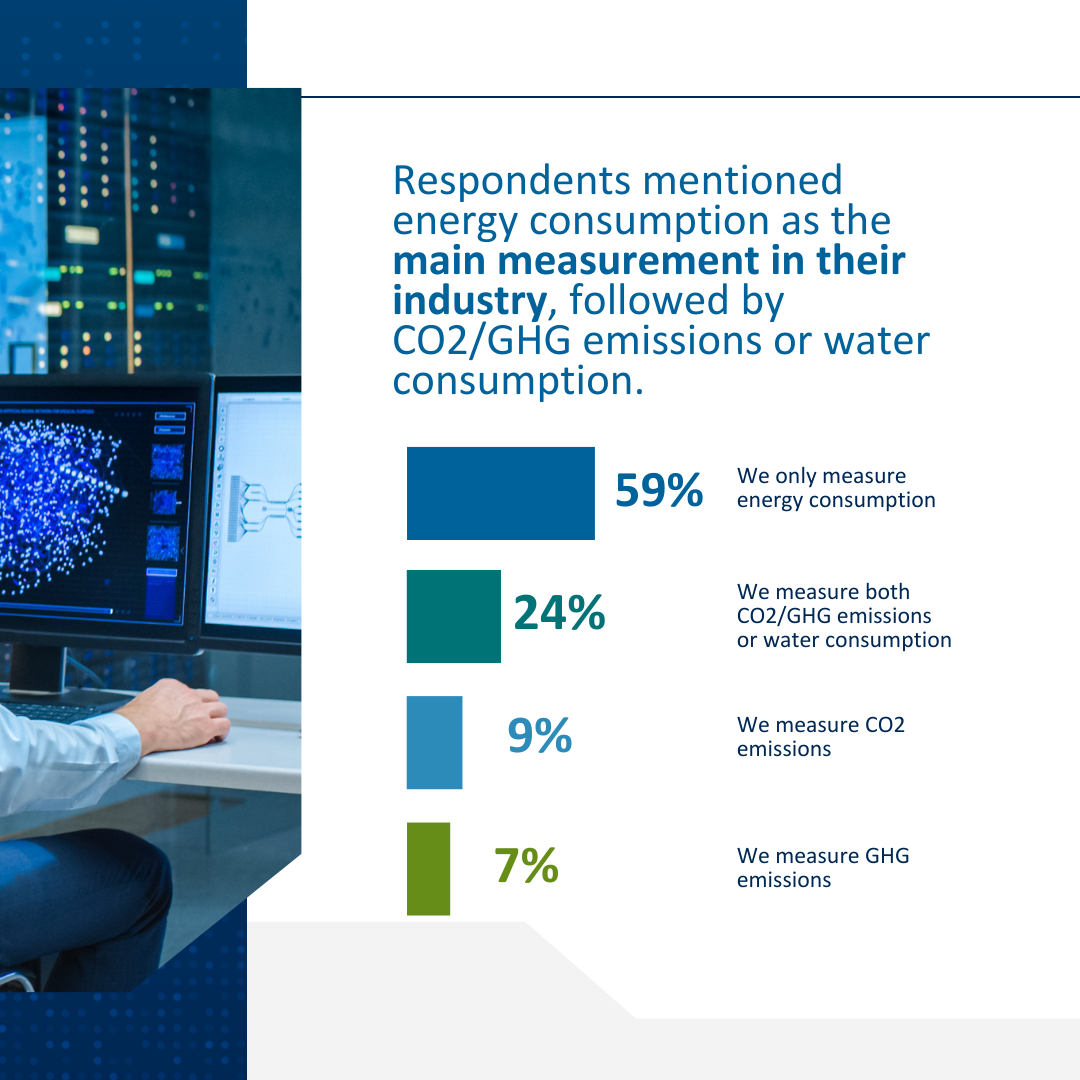
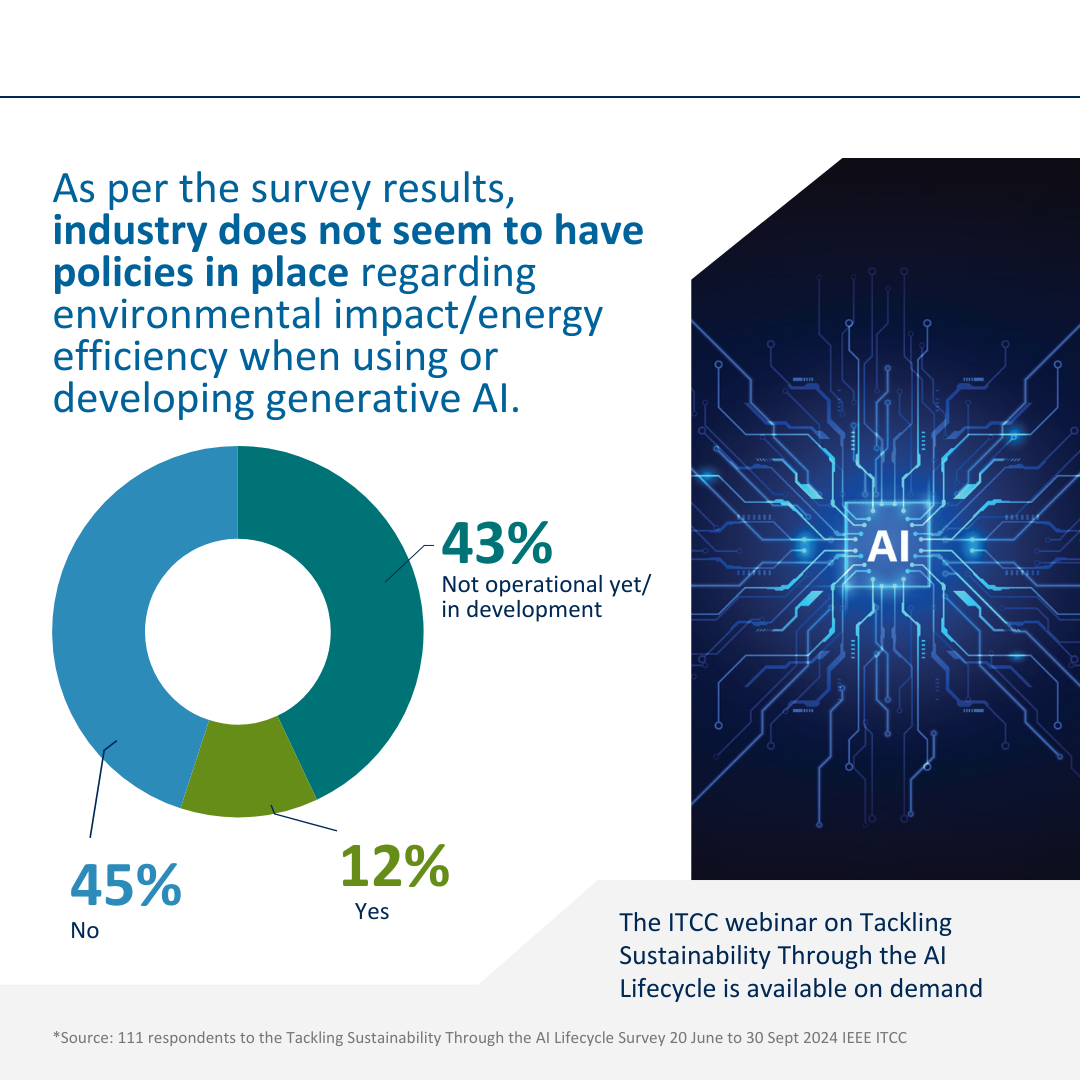
Indeed, the biggest factors weighing in AI deployment decisions are mostly financial, reminds Arti Garg, expert on sustainable data and AI at Hewlett-Packard Enterprise: furthering the goals of organizations, driving business or organizational value; and regulatory/compliance considerations. These may incidentally mitigate their systems’ environmental impact typically in the case of companies opting for hosting models locally for data privacy considerations for example.
Regulations, standards and interoperability: how they affect sustainability
We are seeing AI legislation evolve towards integrating a dedicated environmental risk factor: in the US with the NIST AI Risk Management Framework, and in the EU with the AI Act. These regulations are specifically adding environmental reporting to compliance requirements which are behavioral drivers impacting the way AI is implemented. However, there is still an imbalance between language on voluntary commitments and actual hard law on reporting requirements, leaving more room for potential greenwashing. Moreover, Lee Tiedrich, professor at Duke University points out the related risk of compliance to other regulations, such as privacy, which may lead to less sustainable AI systems. Beyond regulation, soft law such as standards establishes commonly accepted terminology, measurements metrics and methods, and can contribute to improve AI sustainability.
Taking stock and ways forward
We are faced with a dual challenge: users are typically not aware of the efficiency or environmental impact of the AI tools they’re using, while deployers tend to over-integrate AI systems when it is often not crucial. Both of these trends significantly increase AI’s carbon footprint throughout its lifecycle, including water stress and rare materials. Major factors influencing decisions to employ AI are mostly financial, regulatory and compliance considerations, which may only incidentally alleviate its environmental impact. While compliance to regulation and standards may contribute to environmentally sustainable AI systems (e.g. creating consensus about terminology and procedures), when addressing other concerns such as privacy, they may result in more complex and energy-consuming processes. These key takeaways highlight the need for prioritization across stakeholders of proper measurement techniques and metrics for AI sustainability as well as factoring sustainability in building AI systems.
Antoine Glory, Machine Learning Engineer and AI Project Coordinator at CEIMIA – Montreal International Centre of Expertise in Artificial Intelligence.
This Blog post is cross posted with CEIMIA at the following link.
- Our webinar Tackling Sustainability Through the AI Lifecycle explored sustainable AI systems from a lifecycle perspective, focusing on design, software optimization, and other critical components. Part of IEEE webinar series on AI and Sustainability, the event is available to watch on demand here.
Photo: CC-BY (Nidia Dias/Deepmind)
Survey
We’d like to hear from you on this topic!
Name three key enablers in building a sustainable AI from design to deployment?
⬇ Let us know below ⬇